Machine learning method leads to better understanding of the North Atlantic Oscillation
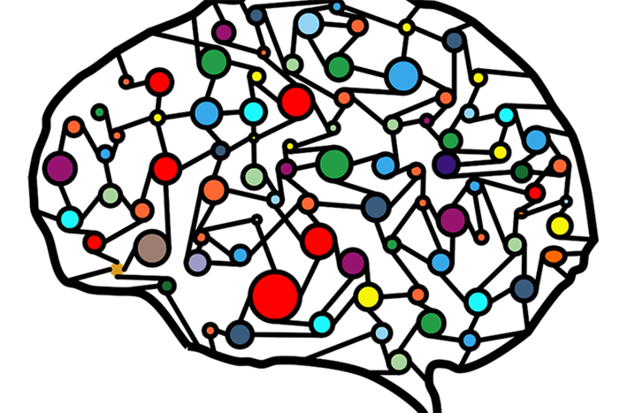
Neural networks may uncover relationships that earlier studies have missed: Pixabay
In a new study at the intersection of climate science and machine learning, research supported by the Climate Program Office’s Modeling, Analysis, Predictions, and Projections (MAPP) Program has recontextualized our understanding of the North Atlantic Oscillation (NAO). The NAO describes the relative strength of high and low-pressure patterns in the Atlantic Ocean. It is an important climate variability pattern in the Northern Hemisphere and it has a strong influence on the weather. MAPP funded Kent State University postdoctoral researcher Chibuike Chiedozie Ibebuchi as part of a larger project to develop monitoring products to characterize temperatures spatially across North America.
Traditionally, scientists used linear techniques to unravel the NAO’s complexities. However, this study, published in Machine Learning: Science and Technology introduces an innovative machine learning approach employing autoencoder (AE) neural networks, known for their prowess in capturing intricate non-linear relationships. The AE method demonstrates remarkable performance, particularly during the winter months, demonstrating an impressive 0.96 correlation with the reference NAO index, surpassing the 0.90 achieved by linear methods.